Understanding how to address dimension mismatches is crucial for accurate t-test results. A dimension mismatch arises when the data being compared in a t-test doesn’t have compatible shapes or sizes. This common statistical error can lead to inaccurate conclusions and invalid analyses. Addressing this issue requires careful data inspection, understanding the specific nature of the mismatch, and implementing appropriate data manipulation techniques. Failing to resolve these discrepancies undermines the validity of the entire statistical inference process. Correctly handling these dimensions is paramount for reliable statistical testing.
Dimension mismatches frequently occur when comparing groups with unequal numbers of observations. For instance, one group might have data for 100 participants, while the other has only 50. This discrepancy directly impacts the statistical power of the t-test and can affect the accuracy of the p-value. Another common scenario involves comparing datasets with different numbers of variables or features. This is particularly relevant in multivariate analyses where each data point might be described by numerous measurements. Inconsistent data types, such as mixing numerical and categorical data without appropriate transformation, also contribute to dimension mismatches, leading to computational errors. These problems necessitate the application of specific pre-processing steps to guarantee a successful t-test.
The consequences of ignoring dimension mismatches are substantial. An erroneous t-test can produce misleading p-values, leading to incorrect conclusions about statistical significance. This can have serious implications, especially in research where decisions are based on the results of statistical tests. In clinical trials, for example, a faulty t-test could lead to the approval or rejection of a treatment based on flawed data analysis. Similarly, in financial modeling, incorrect results might impact investment strategies and portfolio management. Therefore, resolving dimensional inconsistencies is not merely a technicality; it is fundamental to the integrity and reliability of the statistical analysis.
Furthermore, the challenges posed by dimension mismatches extend beyond the immediate impact on the t-test itself. The difficulties can cascade through the entire analytical pipeline, affecting subsequent analyses that rely on the results of the t-test. Downstream applications that use the initial t-test results as input might also produce faulty outputs. This highlights the critical need for proactive and thorough data validation before conducting statistical analyses. Addressing dimension mismatches early in the analytical process ensures the reliability of the conclusions drawn throughout the investigation.
How to address dimension mismatches in t-tests?
The application of a t-test requires the data to be in a specific format. Specifically, the groups being compared must be of consistent dimensions. A common problem arises when comparing datasets of unequal length or with different numbers of variables. This mismatch prevents the statistical software from performing the calculations correctly. The subsequent steps outline a structured approach to resolving these discrepancies. The key lies in careful data preprocessing and validation. Understanding the structure of the data is crucial before proceeding with any analysis.
-
Data Inspection and Cleaning:
Begin by meticulously examining the dimensions of each dataset involved in the t-test. Utilize descriptive statistics and visualizations to identify inconsistencies. Remove or address any missing values or outliers that could contribute to dimension mismatches. Ensure data types are consistent across the datasets. A thorough understanding of the data structure is essential.
-
Data Reshaping:
If the datasets have unequal numbers of observations, consider techniques like data imputation (replacing missing values) or subsampling (reducing the larger dataset to match the smaller one). However, these methods should be applied judiciously, considering their potential impacts on the statistical power and bias. Alternatively, you might investigate using a different statistical test better suited to unbalanced data.
-
Data Transformation:
If different variables exist, decide which variables are truly relevant for comparison. You may need to select a subset of variables to ensure that the datasets have the same number of dimensions. Alternatively, methods like principal component analysis (PCA) might be used to reduce the dimensionality to a common set of principal components.
-
Statistical Test Selection:
In some cases, the underlying problem is not with the data dimensions but rather with the appropriateness of the t-test. If the data violates the assumptions of the t-test (e.g., normality, homogeneity of variances), consider using a non-parametric alternative, such as the Mann-Whitney U test, which is less sensitive to these assumptions and may handle differing data dimensions more flexibly.
Tips for Preventing Dimension Mismatches
Proactive measures to prevent dimension mismatches are far more efficient than corrective actions. A well-structured data management strategy can significantly reduce the likelihood of encountering such issues during the t-test process. Focusing on data integrity and consistency at the initial stages of data collection and processing safeguards the entire statistical analysis workflow. Careful attention to detail ensures a smoother analytical process.
Implementing best practices at each stage of the data pipeline is critical in avoiding dimensionality problems. This includes rigorous data validation, meticulous data cleaning, and consistent record-keeping. Such practices ensure that the data conforms to the requirements of the chosen statistical test.
-
Standardized Data Collection Protocols:
Establish consistent data collection methods to ensure uniform data structures across all datasets. This minimizes variations in the number of observations or variables collected.
-
Data Validation Checks:
Implement automated checks during data entry to flag inconsistencies in data dimensions or data types. This proactive approach minimizes the risk of propagating errors.
-
Regular Data Audits:
Conduct regular audits of the datasets to identify potential dimension mismatches before they impact analyses. This allows for timely corrections and prevents significant setbacks.
-
Careful Data Preprocessing:
Employ rigorous data preprocessing techniques such as cleaning, transformation, and handling missing values before conducting any statistical analysis.
-
Documentation:
Maintain detailed records of the data cleaning and transformation steps undertaken. This ensures reproducibility and facilitates troubleshooting.
-
Using appropriate software:
Leverage statistical software packages that provide robust error handling and warnings about potential dimension mismatches. These tools often offer automated checks and diagnostic messages.
Addressing dimension mismatches is paramount to achieving reliable results from a t-test. These issues, if left unaddressed, can lead to inaccurate conclusions and flawed research outcomes. The systematic approach outlined earlier provides a pathway to navigate these challenges effectively. Each step is designed to ensure data integrity and improve the reliability of the analysis.
The various methods and techniques discussed offer a comprehensive toolkit for handling dimension mismatches. However, the most effective strategy involves a combination of proactive measures to prevent these issues from arising in the first place, coupled with corrective actions when such mismatches are encountered.
Ultimately, resolving dimension mismatches hinges on careful data management and a deep understanding of the statistical principles underlying the t-test. Through meticulous attention to detail and a rigorous approach to data analysis, the validity and reliability of the statistical conclusions can be significantly enhanced. This ensures the integrity and value of the research.
Frequently Asked Questions
Addressing dimension mismatches in t-tests can be challenging, but understanding the underlying causes and applying the correct strategies can greatly improve the reliability of statistical analyses. This section addresses common queries regarding this topic.
-
What happens if I ignore a dimension mismatch and proceed with the t-test?
Ignoring a dimension mismatch can lead to inaccurate p-values and incorrect conclusions about statistical significance. The results will be unreliable and potentially misleading, undermining the validity of your research.
-
Can I use imputation to resolve a dimension mismatch?
Imputation can be used to fill missing values, but it’s crucial to choose an appropriate method (e.g., mean imputation, multiple imputation) and understand its potential impact on the results. Over-reliance on imputation can bias the analysis, so it’s not a universal solution for all dimension mismatches.
-
My datasets have different numbers of variables. How can I proceed?
If your datasets have different numbers of variables, consider feature selection or dimensionality reduction techniques like PCA to reduce the number of variables to a common set before performing the t-test. Alternatively, determine if the additional variables are relevant for comparison; if not, exclude them.
-
What if my data violates the assumptions of the t-test?
If your data violates the assumptions of a t-test (normality, homogeneity of variances), consider using a non-parametric alternative such as the Mann-Whitney U test, which is less sensitive to these assumptions and might be more suitable for your data structure.
-
How can I ensure data consistency across multiple datasets?
Establishing clear and standardized data collection protocols is critical. Utilize data validation checks and conduct regular audits to identify and correct inconsistencies early in the process. Implement automated data checks during data entry to flag discrepancies.
-
What is the importance of documentation in resolving dimension mismatches?
Detailed documentation of the steps taken during data cleaning and transformation is crucial. This ensures reproducibility and assists in identifying the source of the problem if further issues arise. It also enhances transparency and allows others to scrutinize your methodology.
Successfully navigating the challenges of dimension mismatches requires a blend of technical expertise and a rigorous approach to data management. Proactive measures and careful planning can greatly minimize these issues.
The tools and techniques outlined here, when used correctly, offer a powerful means of ensuring accurate and reliable t-test results. A clear understanding of the data structure and the assumptions of the statistical test are paramount.
Ultimately, the goal is to obtain valid and interpretable results, and addressing dimension mismatches is a critical step in that process. The emphasis should remain on sound statistical practice and careful attention to detail throughout the entire analytical pipeline.
In conclusion, effectively addressing dimension mismatches is essential for obtaining accurate and reliable results from a t-test. By carefully inspecting the data, employing appropriate data manipulation techniques, and adhering to good data management practices, researchers can confidently utilize the t-test for valid statistical inference.
Youtube Video Reference:
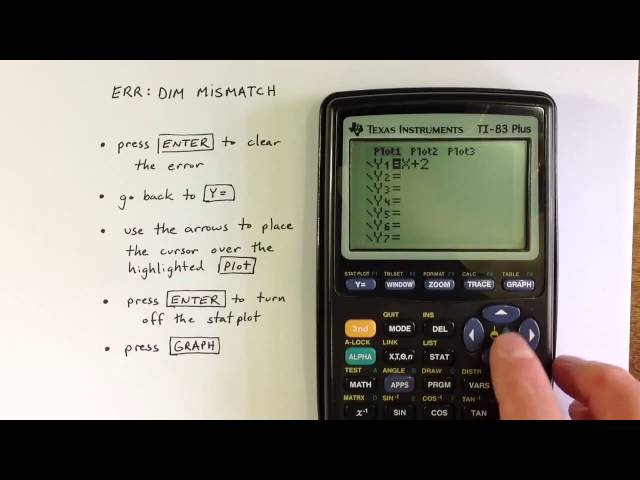