Understanding how to iterate through the elements of a vector is fundamental to many programming tasks. Efficient traversal allows for processing, manipulation, and analysis of vector data, impacting performance and code clarity. This process involves systematically accessing each element within the vector’s defined structure. Various methods exist, each with its own strengths and weaknesses depending on the specific application and programming language. Mastering these techniques unlocks the full potential of vector data structures. The choice of method often depends on whether you need the index of each element or simply need to access the values themselves. Correctly implementing iteration ensures accurate results and prevents common programming errors.
Vectors, as dynamic arrays, store collections of elements of the same data type. Accessing these elements requires a systematic approach, and looping provides the mechanism for this sequential access. The efficiency of the looping method significantly affects program runtime, particularly with large vectors. Consider the memory overhead involved and choose a method that balances speed with memory management. Different programming languages offer various built-in functions or libraries to simplify vector traversal, reducing the need for manual index management. Understanding these tools optimizes development time and code maintainability. The complexity of the loop depends largely on the task at hand; some operations may require simple iteration, while others may necessitate more sophisticated methods.
The choice of iterative method significantly impacts code readability and maintainability. A well-structured loop is easy to understand and debug, while a poorly written one can lead to confusion and errors. Nested loops, for example, may be necessary for multidimensional vector processing but require careful attention to avoid off-by-one errors or infinite loops. Debugging and testing become crucial to ensure the accuracy of the results obtained from vector processing. Consider using debugging tools and techniques to identify and correct any potential issues during development. Proper error handling is vital to prevent program crashes or unexpected behavior.
Furthermore, optimizing vector traversal can lead to significant performance improvements, especially in applications involving large datasets or computationally intensive operations. Understanding the underlying mechanisms of how vectors are stored in memory and how the chosen iteration method interacts with that storage can guide optimization efforts. Careful consideration of data structures and algorithms can improve efficiency. For instance, choosing the right loop structure for a given task can drastically reduce execution time. Profiling and benchmarking techniques can also help identify performance bottlenecks related to vector processing.
How to iterate through a vector?
Iterating, or looping, through a vector involves accessing each element within the vector sequentially. This fundamental operation is crucial for various programming tasks such as data processing, searching, sorting, and manipulating vector contents. The approach depends heavily on the programming language and desired outcome whether index values are needed in conjunction with the elements or only the elements themselves are required. Several techniques are available, offering various trade-offs in terms of readability, efficiency, and complexity. Selecting the appropriate technique is a critical step in writing efficient and effective code. Understanding the underlying principles of memory management and access patterns is essential for optimal performance.
-
Step 1: Initialize a loop counter.
Before beginning the iteration, a counter variable is typically initialized. This variable will track the current position within the vector. The initial value is often 0, corresponding to the first element’s index (assuming 0-based indexing, common in many languages).
-
Step 2: Set the loop termination condition.
The loop continues until a specified condition is met. This condition usually involves comparing the counter variable against the vector’s size. The loop terminates when the counter exceeds the last valid index of the vector. Failing to define this correctly can lead to runtime errors or unexpected behavior.
-
Step 3: Access vector elements.
Inside the loop body, the current vector element is accessed using the loop counter as an index. For example, in many languages `vector[counter]` will retrieve the element at the `counter`th position. This element can then be processed or manipulated as required by the specific algorithm. It’s crucial to ensure the index is within the bounds of the vector to prevent out-of-bounds errors.
-
Step 4: Increment the loop counter.
After processing the current element, the loop counter is incremented to move to the next element. This ensures that all elements in the vector are eventually processed. The increment value is typically 1, moving sequentially through the vector. The manner in which the loop counter is incremented (e.g., `counter++`, `counter += 1`) is language-specific.
Tips for Efficient Vector Traversal
Optimizing the process of accessing and processing data within a vector can significantly improve the performance of applications dealing with large datasets. Understanding the underlying data structures and algorithms can lead to significant gains in efficiency. Choosing the right approach is often determined by the specific task at hand and the programming language being utilized. Simple changes can significantly impact program speed and resource consumption.
Careful planning and consideration of alternative methods can lead to substantial improvements in overall program performance and maintainability. The choice of iteration method should not be made in isolation but rather in the context of the broader algorithm and application needs. By understanding the various techniques available and their respective strengths and weaknesses, programmers can make informed decisions that impact overall program efficiency.
-
Use iterators:
Many programming languages provide iterators that abstract away the details of index management, making the code cleaner and often more efficient. Iterators simplify the process of traversing vectors, reducing the risk of off-by-one errors or other common index-related issues. They provide a higher-level interface that facilitates simpler and more readable code.
-
Avoid unnecessary copying:
Copying entire vectors repeatedly can consume significant memory and time. Where possible, work directly with the original vector to avoid unnecessary overhead. Efficient processing focuses on minimizing data movement and duplication. Passing vectors by reference (where supported by the language) is a key strategy.
-
Consider parallel processing:
For very large vectors, parallel processing can significantly speed up the iteration process by distributing the workload across multiple cores. Techniques like multithreading or multiprocessing can drastically reduce computation time, particularly for CPU-bound tasks. The level of parallelization depends on the task and hardware resources.
-
Optimize inner loops:
If nested loops are used, optimizing the inner loop is often more impactful. Even small improvements in the inner loop can lead to significant performance gains overall, due to the repeated execution of this code. Focus on reducing the number of operations inside the inner loop.
-
Choose the right data structure:
If possible, consider using more efficient data structures for specific tasks. For example, if the task involves frequent searching, a hash table might be preferable to a vector. The suitability of a particular data structure is directly related to the type of operations performed on it.
-
Pre-allocate memory:
If the vector’s size is known in advance, pre-allocate the memory needed to avoid repeated memory allocation during iteration. This avoids the performance overhead of dynamic memory allocation, leading to faster processing. This is particularly beneficial for large vectors.
-
Use appropriate algorithms:
Select algorithms that are well-suited to the task and minimize the number of vector accesses. Algorithm design is crucial for overall efficiency. Well-chosen algorithms reduce the computational load and improve the overall performance of the code.
Efficient vector traversal is essential for a range of applications. From simple data analysis to complex machine learning algorithms, the ability to quickly and efficiently process vector data is a cornerstone of effective programming. The strategies discussed above can significantly improve processing speeds and reduce computational costs, especially when dealing with large volumes of data.
Careful code design and optimization is crucial for creating robust and efficient programs. The choice between different looping methods is not merely a matter of syntax but also profoundly impacts the overall performance and resource usage of the application. Understanding these nuances allows developers to make informed decisions leading to well-optimized code.
In summary, proficiency in vector traversal techniques is a key skill for any programmer. By mastering various approaches and applying optimization strategies, developers can build high-performance applications that effectively handle large datasets. Regular code review and profiling can identify further optimization opportunities and ensure that the code remains efficient over time.
Frequently Asked Questions about Vector Traversal
Several common questions arise concerning iterating through vectors, reflecting the importance of this fundamental programming task. Understanding these common issues clarifies the techniques and best practices required for accurate and efficient code.
-
How do I handle vectors of different sizes?
The loop termination condition should always be based on the size of the specific vector being processed. Using a fixed loop count can lead to errors if the vector size varies. Dynamically determining the vector’s size and using that as the termination condition ensures robust handling of vectors with different lengths.
-
What are the consequences of accessing an element outside the vector’s bounds?
Accessing elements outside the valid index range of the vector typically results in a runtime error. This can cause the program to crash or produce unpredictable results. Careful indexing is crucial to prevent such errors. Robust error handling can mitigate the impact but prevention is always better than cure.
-
How can I efficiently search for a specific element within a vector?
Linear search is simple but inefficient for large vectors. More sophisticated methods such as binary search (for sorted vectors) or hash tables can dramatically improve search efficiency. The optimal search method depends on the characteristics of the data and the frequency of searches.
-
How does vector traversal differ between languages like C++, Python, and Java?
While the underlying concept remains the same, the implementation differs. C++ uses iterators frequently, Python offers list comprehensions and for loops, and Java uses enhanced for loops and iterators. Each language provides specific tools to simplify the process of accessing vector elements.
-
What are some common errors encountered when iterating through vectors?
Off-by-one errors, infinite loops, and out-of-bounds accesses are common pitfalls. Careful attention to loop counters, termination conditions, and index values is essential to prevent these errors. Thorough testing and debugging help identify and rectify such issues.
-
How can I optimize vector traversal for performance-critical applications?
Use appropriate data structures, avoid unnecessary copying, leverage parallel processing where possible, and choose efficient algorithms. Profiling the code helps identify performance bottlenecks and guide optimization efforts. Understanding memory access patterns is also crucial.
Mastering efficient vector traversal is crucial for creating high-performance applications. The strategies and considerations outlined here aim to equip programmers with the necessary knowledge for handling vector data effectively.
Careful consideration of the nuances of vector traversal leads to efficient and robust code. The choice of method, combined with optimization techniques, dramatically impacts the overall performance of the application. Continuous learning and code review are essential for maintaining optimal performance.
In conclusion, understanding how to effectively traverse a vector is a fundamental skill in programming. The techniques presented here provide a solid foundation for tackling various challenges related to vector data processing. Remember to choose the most appropriate method based on the specific task and consider optimization strategies for performance-critical applications.
Ultimately, mastering how to efficiently iterate through a vector is essential for building robust and high-performing applications across numerous programming domains. Continuous learning and attention to detail are key to success in this crucial aspect of programming.
Youtube Video Reference:
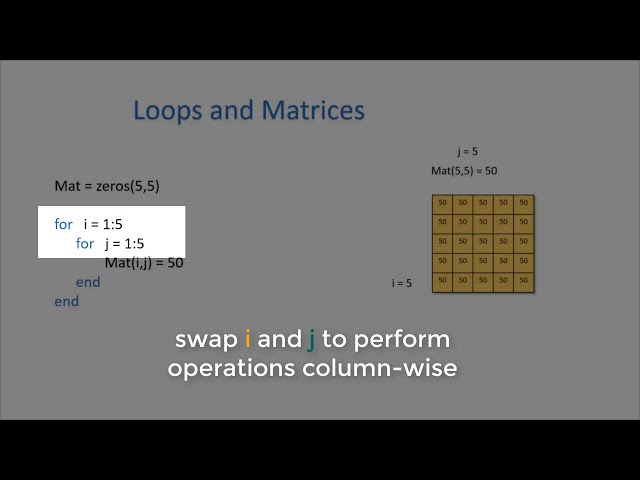